Example: Ornstein_Uhlenbeck
Ornstein-Uhlenbeck process
Figure 2: Two realizations of the Ornstein-Uhlenbeck process for parameters τ=1.0 and σ=0.1 (black curve), and for τ=0.1 and σ=0.31622 (red curve). In both cases the noise intensity is σ^2*τ=0.01 . The red curve represents a noise that more closely mimics Gaussian white noise. Both realizations begin here at x(0)=1.0 , after which the mean decays exponentially to zero with time constant τ.
Andre Longtin (2010) Stochastic dynamical systems. Scholarpedia, 5(4):1619.
Sebastian Schmitt, 2022
import matplotlib.pyplot as plt
import numpy as np
from brian2 import run
from brian2 import NeuronGroup, StateMonitor
from brian2 import second, ms
N = NeuronGroup(
2,
"""
tau : second
sigma : 1
dy/dt = -y/tau + sqrt(2*sigma**2/tau)*xi : 1
""",
method="euler"
)
N.tau = np.array([1, 0.1]) * second
N.sigma = np.array([0.1, 0.31622])
N.y = 1
M = StateMonitor(N, "y", record=True)
run(10 * second)
plt.plot(M.t / second, M.y[1], color="red", label=r"$\tau$=0.1 s, $\sigma$=0.31622")
plt.plot(M.t / second, M.y[0], color="k", label=r"$\tau$=1 s, $\sigma$=0.1")
plt.xlim(0, 10)
plt.ylim(-1.1, 1.1)
plt.xlabel("time (sec)")
plt.ylabel("Ornstein-Uhlenbeck process")
plt.legend()
plt.show()
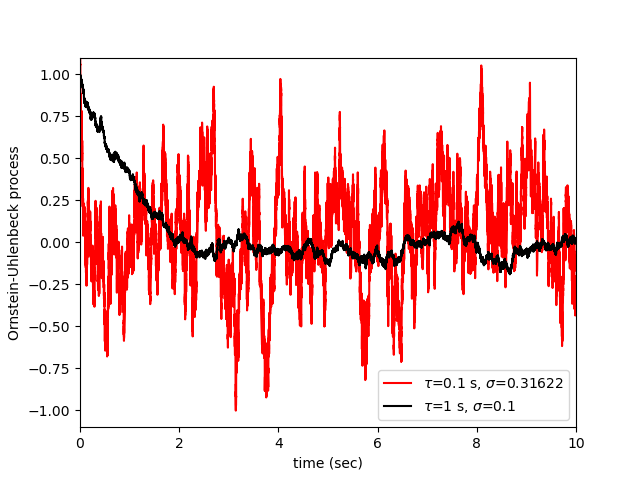